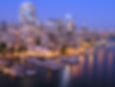
Before a comprehensive breakdown of what the results of the analyses mean can be made, it is first necessary to understand and take into account the limitations of the data and the tools available. In terms of data provenance, the crime dataset provided through the OpenData catalogue does not represent the actual locations of a specific criminal activity – rather, locations for property crime were provided within the ‘hundred block’, within the ‘general area’ of the block. What this means is uncertain but it likely means that the locations represented in the datasets have been randomized or pseudo-randomized and that some of the patterns observed in their spatial distributions may be the result or have otherwise been influenced by a ‘non-real’ process. Some of the circular artifacts of ‘no pattern’, for example, in the results of the hotspot analysis, may be the result of an algorithm generating random or pseudo-random points around a ‘real’ location based upon a fixed distance over the course of years, thereby creating an ‘island’ of ‘no pattern’-ness in a larger ‘sea’ of cooling or heating trends.
​
Emerging Hotspot Analysis
​
For the all property crime, break-and-enter and vehicle theft maps, the majority of downtown was classified as persistent hot spots, while much of the most affluent suburbs outside of the core were classified as persistent cold spots from 2007 to 2017. A potential explanation for these consistent visual trends may be the distribution of population density throughout the city, and the limitations of the emerging hotspot analysis. The underlying population density of Vancouver is not homogenous throughout– the downtown core quite clearly houses more individuals per unit area than the single-family oriented suburbs surrounding it. Because the emerging hotspot analysis did not account for these densities, the greater occurrences of crimes in the more densely-populated regions of the city would likely result in hot spots being identified in these areas, while cold spots in the suburbs would be identified due to the lower abundance of crime occurrences, even if per-capita crime rates may not differ as dramatically. Potential future analysis with this dataset may include methods such as kernel dual-surface estimates, which would allow for the creation of probability surfaces for these categories of property crimes while also accounting for the underlying population densities that the point data resides upon.
In the case of the all property crime map, a large region of intensifying hot spots was identified around the Mount Pleasant area of the city. A potential explanation for this observed phenomenon could be the geographic nature of the offenders in Vancouver. If many of the perpetrators of property crime are ‘marauders’– where they travel further away from their homes in order to commit crime– the Mount Pleasant area would be a prime candidate for individuals to travel to, due to its ready accessibility via skytrain or major commuter bus lines such as the 99 B-line (Paulsen, 2007). The availability of transit may also play a role in the intensification or persistence of cold spots in the western and southwestern portions of Vancouver. With no skytrains and fewer major bus lines travelling towards Point Grey and Shaughnessey, potential marauders may have reduced means to travel to these areas and commit offenses. Future research should thus incorporate geographic profiling into the study of how property crime is changing in Vancouver over time, in order to better identify patterns and assist law enforcement.
​
​
Maximum Entropy Crime Suitability
​
During the initial run of the Maxent model an interesting bias was observed in the outputs, whereby the ‘distance to street’ variable was ranked as disproportionately important in assigning the locations of the crime occurrence dataset. With hindsight, the reasoning for this was obvious – in a dense city environment like Vancouver, there are comparatively few locations within the scope of the study that are not within a few hundred metres of a public street. When this variable was placed in Maxent, the model therefore evaluated the crime occurrences and found that they were all very close to a street – and therefore ranked it as a much higher factor than was likely appropriate. The ‘distance to street’ variable was therefore excluded and the Maxent model run again, producing the figures seen in the Results section and which provided a much more informative set of data.
This exclusion has important implications for the construction of the ‘crime distribution’ model created through Maxent. In a large scale analysis of predictor variable types used in Maxent species models Bradie and Leung (2016) found that ‘structural’ variables like precipitation, temperature, distance to water, habitat patch and bathymetry were consistently important factors across a large sample of species across many families. These factors operate on large scales and help determine the ‘structure’ of the terrestrial or aquatic landscapes they interact with. The intention of including the grid structure of the city (ie including ‘distance to street’) as a variable was for it to act as a similar baseline principle for the ‘crime distribution’ model, but the provenance of the data provided and the nature of how cities are structured makes it untenable. The success of factors like distance from schools or distance from transit stations as predictors makes incorporating more complex socioeconomic indices a feasible potential future path for research. Metrics like Bell et al.’s VANDIX (Vancouver Area Neighbourhood Deprivation Index), constructed out of census data and health records, may represent a more robust and empirically-sound method of assessing socioeconomic status than trying to develop proxies like property value or distance to amenities that may have more difficulty capturing nuance in distributions that a targeted schema may more successfully quantify.
More complex metrics like VANDIX may also have the benefit of avoiding the pitfalls of the spatial distributions of the identified factors biasing the prediction of the model. The shelter dataset for example is heavily biased towards the downtown area, and has a strong permutation importance value for crime datasets that are similarly clustered downtown like commercial break-and-enters and ‘other theft’, which includes stolen personal items. In contrast, ‘distance from school’ may also have reached its place as second strongest predictor variable due to the evenness of its distribution across a landscape – any given crime occurrence is not that far from a school not because the school is contributing factor to the crime, but because schools are common enough and widely distributed enough that there are few places in the study site that aren’t far from a school.
The direction of future analysis is, in the light of these spatial distribution factors and the availability of more complex and comprehensive metrics like VANDIX, clear. To begin with a solid foundation upon accurately georeferenced crime data must be sourced from the City of Vancouver or Vancouver Police’s databases. Having confidence in the georeferencing of the initial training sets is important for deriving information from finer spatial resolutions, doubly so when attempting to determine the potential causes of crime from their spatial relationships. Thus, finding variables with stronger explanatory power should be the next logical step. Variables such as ‘Euclidean distance from [x] feature’ may be too simplistic for developing truly predictive models, and more complicated derivative products, like calculated distance along a transit network or from identified transit hubs, may be useful tools in future analyses.