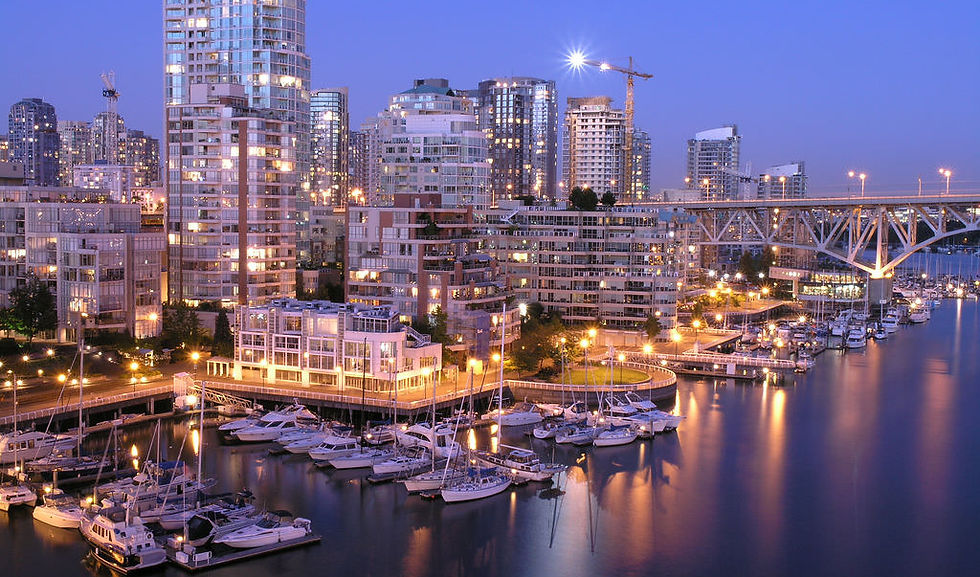
Abstract
​
Rapid development and expansion of the city of Vancouver has subsequently led to changes in the landscape of property crime around the city. Understanding spatiotemporal trends and creating predictive models are necessary in order to better inform policymakers and authorities on best practices for crime management and public safety. Through an emerging hotspot analysis from 2007-2017 for a number of property crime variables, emerging and persistent hot spots were identified in the downtown core and in the Mount Pleasant region, while emerging and persistent cold spots were identified in south and southwest Vancouver, which may be due to underlying population densities and criminal activity patterns interacting with existing transit infrastructure. Using a Maximum Entropy model and 2017 property crime data, proximity to homeless shelters was found to be an important predictive variable for the occurrence of crime, which may be attributed to the density of shelters in downtown. Schools were also found to be an important predictor, which may be an artifact of their relative even dispersal across the landscape of Vancouver. The relative importance of each of the socioeconomic variables incorporated into the model was also dependent on the type of property crime being examined, which may highlight important underlying factors that could be incorporated in future analyses.
​
​
Introduction
​
Vancouver is one of North America’s fastest growing cities, and with this growth comes changes in the prevalence and distribution of crime throughout the city. It is important for civic leaders, law enforcement and other authorities to understand the trends present in these changes in order to best adapt to changing socioeconomic dynamics. There are many methods available, however, to examine these trends in crime and how they might relate to a changing social environment, and this study will utilize hotspot analysis to quantify past changes in the distribution of crime (Andresen, Linning, & Malleson, 2017; Andresen, 2007; Andresen, 2006).
This study will also seek to adapt the ‘MaxEnt’ maximum entropy modelling software commonly used in landscape ecology to predict potential trends in the future based upon identified socioeconomic or spatial factors. This software and method has been identified as being useful for the problem of predicting crime because of the similarities present in the types of data used in both analyses. MaxEnt is used in circumstances where only occurrences are known and not absences, and various potential landscape factors like precipitation or vegetation type are used as constraints on the ‘suitability’ of the study area for the organism (Andrew, Chandler, Yackulic & Nichols 2012). In crime, similarly, only occurrence points are known and the model would similarly seek to use spatial factors like distance from streets as constraints on the potential ‘suitability’ for a given location to play host to an act of criminality.
In order to narrow the scope of these analyses, property crime has been identified as an ideal subset through which to test the parameters of the methods proposed here, as property theft represents a large data set with a number of potential subcategories that allowed for nuance in the factors that may drive them.
​